Parenting a 3-Year-Old Robot: CMU and Meta AI Researchers Unveil RoboAgent
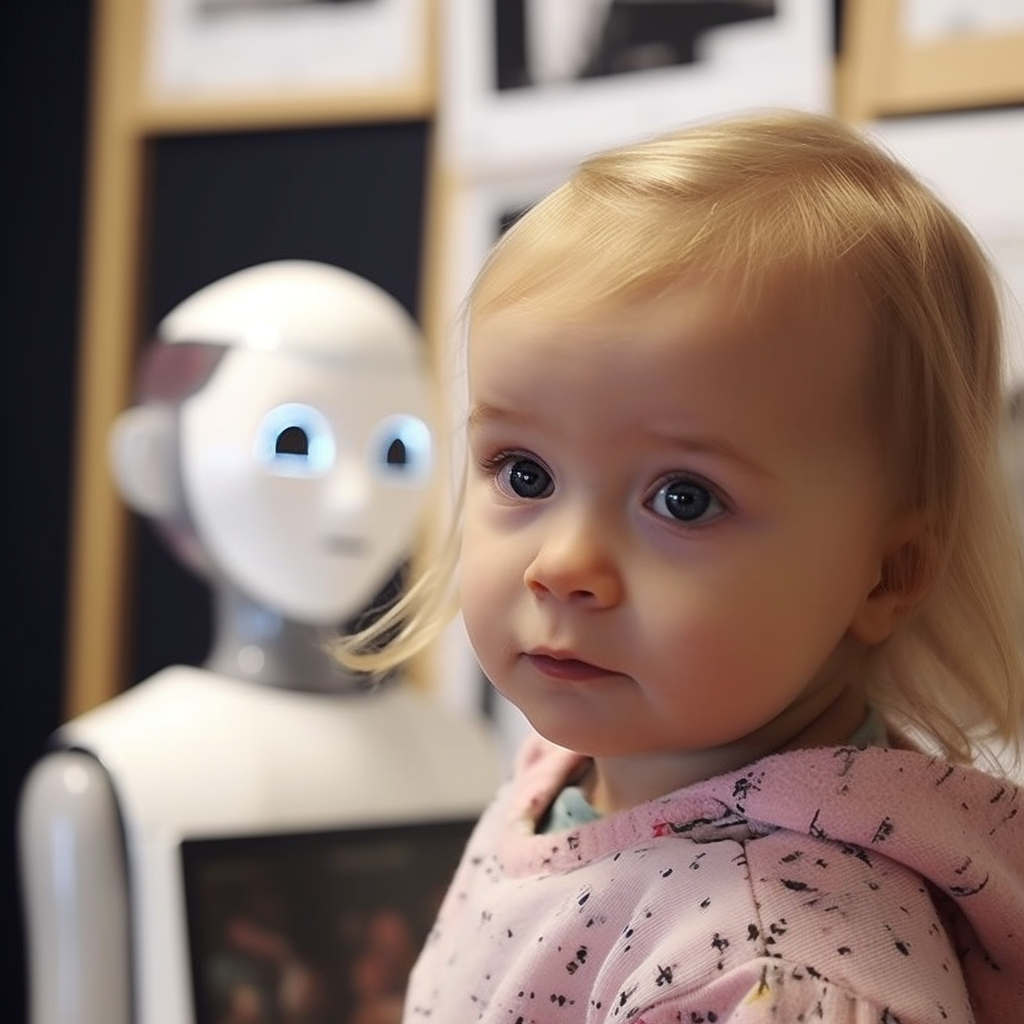
In a groundbreaking collaboration, researchers from Carnegie Mellon University (CMU) and Meta AI have introduced RoboAgent, an artificial intelligence agent that mirrors the learning abilities of a 3-year-old child. Inspired by the way infants learn by observing and imitating, this innovative project aims to imbue robots with the capacity to simultaneously master multiple skills and adapt them to various unseen scenarios.
RoboAgent represents a significant advancement toward the creation of versatile robotic agents capable of learning efficiently, effectively navigating novel situations, and expanding their skill set over time. Unlike the current paradigm of highly specialized robots designed for singular tasks, RoboAgent embodies a holistic learning approach inspired by human cognition.
Vikash Kumar, an adjunct faculty member in CMU's School of Computer Science's Robotics Institute, emphasized the unique approach of RoboAgent. "Current robots are highly specialized and trained for individual tasks in isolation. In contrast, we set out to create a single artificial intelligence agent capable of exhibiting a wide range of skills in unseen scenarios. RoboAgent learns like human babies — leveraging a combination of abundant passive observations and limited active play."
The agent's capabilities are indeed remarkable. RoboAgent has demonstrated the ability to accomplish 12 manipulation skills across varying scenarios. What sets this research apart is its implementation in real-world environments rather than simulations, all while utilizing significantly less data than prior endeavors. This opens the door to a robotic learning platform that can adapt seamlessly to changing surroundings.
Abhinav Gupta, an associate professor in the Robotics Institute, highlighted the impressive complexity of skills achieved by RoboAgent. "We've shown a greater diversity of skills than anything ever achieved by a single real-world robotic agent with efficiency and a scale of generalization to unseen scenarios that is unique."
RoboAgent's learning process combines self-experiences and passive observations from internet data, much like how a parent guides a child. Researchers teleoperated the robot through tasks to provide it with relevant self-experiences. Its unique policy architecture enables reasoning even with limited experiences, allowing the agent to predict and aggregate decisions based on temporal chunks of movements.
Importantly, RoboAgent learns from online videos, mirroring how infants acquire knowledge by observing their surroundings. This approach helps the agent understand human interactions with objects and various skills required to complete tasks successfully. This knowledge is then applied to tackle unknown tasks or uncharted environments.
Shubham Tulsiani, an assistant professor in the Robotics Institute, sees RoboAgent as a significant step toward versatile robots that can function effectively across diverse settings. "RoboAgent can quickly train a robot using limited in-domain data while relying primarily on abundantly available free data from the internet to learn a variety of tasks. This could make robots more useful in unstructured settings like homes, hospitals and other public spaces."
The team's commitment to open-source collaboration further underscores their dedication to the field's advancement. Their trained models, codebase, hardware drivers, and comprehensive dataset collected during this research are available to the public. RoboSet, the dataset, is the largest publicly available robotics dataset on commodity hardware, aiming to foster the growth of a foundational general robotic agent.
As the world of robotics progresses into new dimensions, the RoboAgent project signals an exciting leap toward more adaptable, capable, and versatile robots. The merging of AI and human-inspired learning methodologies sets the stage for robots that can continually evolve their capabilities and navigate diverse environments, further blurring the line between artificial and human intelligence.